Causal AI Use Cases
Causal AI has the potential to transform healthcare by analyzing vast amounts of data to accelerate drug discovery, personalize treatments, and improve clinical trials.
As health data continues to grow exponentially, the role of Causal AI in uncovering actionable insights is becoming increasingly significant.
1
Causal Inference for Drug Repurposing
Causal AI techniques can be used to identify existing drugs that may have new therapeutic uses. By understanding the causal relationships between drug mechanisms and disease pathways, AI models can analyze large healthcare datasets (e.g., clinical trials, patient records) to predict which approved drugs could be effective for other, under-researched conditions. This approach accelerates the drug discovery process by leveraging existing safety and efficacy data.
Example: Using causal models to predict which cardiovascular drugs might inhibit tumor growth in specific cancers, such as repurposing beta-blockers to target mechanisms associated with metastasis.
2
Personalized Treatment Plans via Causal Models
Causal AI can help in developing personalized treatment plans for patients by modeling the complex interactions between patient characteristics (genetic data, medical history, lifestyle) and the effects of various treatments. This can be especially helpful in areas like oncology or rare diseases where standard treatment protocols may not be effective for everyone, or in therapeutic areas with high unmet needs where no treatments exist. By understanding the underlying causal relationships, AI can help tailor treatments to individual needs, no matter how rare.
Example: A causal AI model analyzes how a specific cancer drug will interact with a patient's genetic profile, predicting optimal dosage and therapy combinations for better outcomes.
3
Clinical Trial Optimization
Clinical trials are expensive and time-consuming, often facing issues like patient dropout and slow recruitment. Causal AI can help in the design and execution of clinical trials by identifying the causal factors that influence patient responses to treatments. This can improve trial recruitment strategies, optimize dosing schedules, and better predict the outcomes of various interventions.
Example: Using causal modeling to identify which demographic or genetic factors increase the likelihood of successful responses to a drug, ensuring more efficient patient selection and more accurate trial outcomes.
4
AI-Powered Alternatives to Animal Testing in Drug Development
With advancements in AI, in silico simulations, and human-centric models, it's becoming increasingly feasible to skip or minimize the use of animal models in the early stages of drug development. AI can be used to simulate drug behavior in human tissues, predict molecular interactions, and optimize clinical trial design—all while using in vitro or computational models that closely mimic human biology. This approach reduces ethical concerns, accelerates the drug development process, and improves the relevance of preclinical data.
Example: AI models that simulate how drugs interact with human receptors or AI-driven organ-on-a-chip technologies allow researchers to test drug efficacy and toxicity in human-like environments, bypassing the need for animal trials. This not only supports ethical considerations but also improves the predictive accuracy of results in humans, potentially leading to faster and more targeted drug development processes.
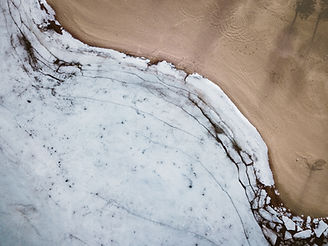